LEARN ABOUT OUR PROJECTS
- Turkana Health and Genome Project
- Life Under Stress
- It's the Microbiome...
- Variance Analytics
- Systems Genetics: Complex Traits
Do modern diseases arise from genomes living in the past?
In a blink of evolutionary time, humans have explored every corner of this planet and have shown an amazing capacity to adapt to extreme conditions. The Turkana, a seminomadic pastoralist tribe, live in northern Kenya in one of the most arid environments in the world. Having retained their traditional lifestyle, the Turkana provide a rare opportunity to address how ecological pressures and natural selection shape human genetic variation (a question we will explore using whole genome sequencing). Further, because of recent infrastructure developments, many Turkana are moving away from their ancestral lands and into cities. This unique situation allows us to ask another important question: what happens when a locally adapted population is transplanted to a novel urban environment? Such urban-rural migrations are commonly accompanied by an increased risk of chronic diseases; yet, our mechanistic understanding of how these transitions impact health is limited. To address this gap, we are contrasting transcriptomic and phenotypic data collected from traditional Turkana versus those that have moved, within their lifetime, to major cities. Together, this work not only allow us to retrace human evolutionary history, but to understand how the disruption of locally adapted systems may lead to disease.
About the Turkana people
The Turkana people inhabit one of the most arid ecosystems in East Africa, with year-round highs of 100F and low levels of seasonal, unpredictable rainfall. The Turkana are pastoral nomads, and 80% of their diet is derived from milk or other animal products. Daily protein intake is thus extremely high (300% of the WHO requirements), but total caloric intake is low (1,300-1,600 kcal/day for adults). Turkana are consequently very lean, yet they undertake the arduous task of collecting water on a daily basis. This process typically involves walking several kilometers (5 to 10 km is not unusual) to wells dug in dry river beds, and hauling water up from the bottom of a well (which can exceed 30 feet during the dry season). Water must then be carried back to the home and shared among family and livestock. As a result, the Turkana drink relatively little water on a daily basis, while tolerating extreme heat and exerting considerable energy; they do so despite limited caloric reserves and a protein-rich diet, which takes considerably more energy to digest than fats or carbohydrates. This extreme lifestyle has likely selected for numerous physiological adaptations in the Turkana people that we aim to uncover.
We are very fortunate to work with amazing team based at the Mpala Research Center under the directorship of our collaborator Dr Dino Martins.
Why do some individuals appear to be more sensitive than others to environmental perturbation?
The answer to this question has broad implications ranging from our ability to make predictions about disease risk from genotype, to our ability to identify the drivers of inter-individual variability, and our understanding of toxicity mode-of-action. Exploring the contribution of genotype-by-environment interactions (GxE) to individual variation has been very challenging in humans, where epidemiological studies exploring GxE are generally underpowered, have difficulties quantifying environmental exposure. To address this problem we created a new community resource to study the genetic basis of complex trait variation in Drosophila melanogaster composed of large, synthetic outbred populations. The approach we outline enables us to break away from traditional, and often underpowered approaches that have relied on inbred strains or RILs. With this new and versatile community resource, we can rear thousands of genetically unique flies drawn from a common genetic pool, expose them to a range of different environments and contrast the ensuing genetic architectures
Data we have collected over the past couple of years indicate that differences in individual sensitivity emerge from the disruption of regulatory systems where individuals that are more sensitive to environmental stress have decreased transcriptional robustness for many genes and that this variation in robustness is under genetic control. We have developed an analytical framework to identify context-dependent transcriptional networks and the polymorphisms that control this variation. The impact of mutations that affect environmental sensitivity are heavily dependent on the interaction between genetic background and environmental stress simultaneously. As important as they are, the epistatic interactions associated with variation in penetrance have been notoriously difficult to identify. We are currently developing experiment and statistical approaches aimed at mapping and testing the contribution of such interaction of individual variation in environmental sensitivity.
Can the microbiome influence host evolutionary trajectories?
The microbiome shapes many traits in hosts, but we still do not understand how it influences host evolution. To impact host evolution, the microbiome must be heritable and have phenotypic effects on the host. However, the complex inheritance and context-dependence of the microbiome challenges traditional models of organismal evolution. We take a multifaceted approach to identify conditions in which the microbiome influences host evolutionary trajectories.
We are currently exploring quantitative genetic models to study how microbial inheritance and phenotypic effects can modulate host evolutionary responses to selection. We are particularly interested in how hosts may leverage locally adapted microbes, increasing survivorship in stressful environments. As well as how microbial variation may increase host phenotypic variation, enabling exploration of novel fitness landscapes.
The complex interplay between host and microbial genetic variation is surprisingly understudied. We use a combination of approaches from experimental evolution in Drosophila to ecological sampling in humans across environmental gradients and lifestyle. We aim to incorporating microbial variation in standard evolutionary and quantitative genetics model to better understand how phenotypic variation is generated, and subsequently, how selection operates across ecological and evolutionary scales.
It is well established in quantitative genetics that stressful environmental exposure tends to increase the phenotypic variance of a population, but how and why?
This is a fundamental question for any biologist interested in understanding the genetic basis of variation for complex traits. Although studies of development, morphology and animal breeding have long noted the heterogeneity of variance among genotypes, this axis of variation has received little attention compared to the effect of genetic variation on trait means. There is now clear evidence for the importance of genetic control of variance and that variance itself is a quantitative trait. This has important implications both in medical genetics and evolutionary biology. If different genetic? backgrounds differ in their? propensity for phenotypic variability, then individuals derived from a high-variability genetic background may exhibit an extreme phenotype by chance alone. A property of that genotype that would not have been informed by traditional mean focused quantitative genetic approaches. In the context of evolutionary change, this could accelerate or slow down adaptation to new conditions. With respect to health, this could result in disease, changes in variance can affect the probability of individuals finding itself in the tails of the distribution. Therefore, by focusing primarily on the effect of genetic variation on trait averages and ignoring its effect on variance, we may be missing a very important axis contributing to phenotypic variation.
Our lab explores this problem both from an evolutionary perspective asking: under what scenario might variance control evolve? What evolutionary forces maintain variation for alleles controlling phenotypic variability? And from a medical perspective: how does variance control affect our ability to make predictions from genotype to phenotype? Does the presence of variance increasing alleles increase the probability an individual being in the tail of distribution?
Collaborators:
Evolutionary dynamics of variability
Benjamin de Bivort Lab (Harvard)
Barbara Engelhardt (The BEE Lab)
Robustness As A Driver Of Disease Emergence
Paivi Pajukanta Lab (UCLA)
Noah Zaitlen Lab (UCSF)
A major focus of the lab is to study how genotype-by-genotype and genotype-by-environment interaction modulates gene regulatory networks and ultimately shape individual variation.
The current quantitative genetic paradigm is driven by a prevailing view that additive genetic models – focused on the mean effect of alternative alleles – adequately explain variation for most phenotypes. Unfortunately, a decade after the popularization of GWAS and in spite of much effort, we have fallen short of the goal of explaining most of the heritability for complex traits in terms of allelic effects. This averaging approach is designed to describe the mean effect of an allele randomized over a large number of the genetic backgrounds and environments. But each individual has faced a unique trajectory of environmental insults, some of which may have quite large genotype-specific effects.
A fast-growing body of evidence indicates that the genotype-phenotype map is much more complicated than Fisher’s additive model would predict. When measurements can be made with reasonable control of the environment, complex, non-additive interrelationships between loci appear to be the rule and not the exception. Furthermore, these allelic effects are often environmentally sensitive. The paradigm derived from traditional quantitative genetics is at odds with a major goal of genetics as we often seek to understand the causal path from genotype to phenotype for individuals and not populations.
In Drosophila, we have developed a unique resource for mapping variation in complex traits using large synthetic Drosophila outbred populations. These genetically diverse mapping panels allow us to control genetic background and allele frequency as well as the environment of each population. Notably, our method breaks away from traditional approaches that often rely on problematic inbred strains. This allows us to rear thousands of genetically unique flies, drawn from a common genetic pool, expose them to different environments and study the combined effect of genetic background and environment perturbation. We are currently focusing on metabolic traits.
In humans, we are collaborating with the lab of Dr Paivi Pajukanta at UCLA and are taking a systems genetics approach to the study of metabolic syndromes (METSIN cohort). Our laboratory has developed fully automated approaches to perform transcriptional profiling at high-throughput for a fraction of the cost of currently available methods. This is allowing us to profile a large number of individuals and use a systems genetics approach to study metabolic variation, echoing our work in flies.
Understanding the genetic basis of complex traits demands that we go beyond describing the relationships between polymorphic DNA and phenotypic variation. To that end we take a system genetics approach, simultaneously measuring variation at multiple levels of biological organization is a necessary first step. Patterns of transcriptional correlation allow the construction of co-expression networks describing how genetic variation affects transcriptional variation (i.e. eQTL), and how directed transcriptional networks in turn correlate with phenotypic variation. Together, this information will allow us to draw the causal path from variation in allele frequency to a phenotypic differences between individuals. Such directionality indicates the flow of biological information and sets the framework through which perturbations can be predicted. This is the promise of systems genetics – the formulation of causal predictions painting a detailed picture of a dynamic genotype-phenotype map.
LEARN ABOUT OUR PROTOCOLS
TM3’seq: a tagmentation-mediated 3’ sequencing approach for improving scalability of RNA-seq experiments
RNA-seq has become the standard tool for collecting genome-wide expression data in very diverse fields, from ecology and developmental biology to quantitative genetics and medical genomics. However, RNA-seq library preparation as well as its sequencing requirements are still prohibitive for many laboratories, in particular when large sample sizes are involved. Recently, the field of single-cell transcriptomics has been able to reduce costs and increase throughput by adopting an approach that barcodes individual samples during reverse transcription and pools them before cDNA synthesis, effectively processing a single sample for most of the library preparation procedure. In contrast, RNA-seq protocols where each sample is processed individually are significantly more expensive and lower throughput than single-cell approaches. Yet, many experimental approaches are designed around follow-up experiments on a subset of samples, and therefore require that individual libraries are generated for each sample. In order to fill this gap, we have developed TM3’seq, a 3’-enriched library preparation protocol that uses Tn5 transposase and preserves the sample identity at each step. TM3’seq is designed for the high-throughput processing of individual samples (96 samples in 6h, with only 3h hands-on time) at a fraction of the cost of commercial kits ($1.5 per sample), while recovering gene expression profiles of the same quality as the commercial kits. We expect that the cost- and time-efficient features of TM3’seq make large-scale RNA-seq experiments more permissive for the entire scientific community.
TM3’seq: a tagmentation-mediated 3’ sequencing approach for improving scalability of RNA-seq experiments Luisa F. Pallares, Serge Picard, Julien F. Ayroles. (2019) bioRxiv https://doi.org/10.1101/585810
LEARN ABOUT THE LAB
Julien Ayroles
Principal Investigator
Julien has taken a diverse path throughout his career. As an undergraduate at the University Paul Sabatier in Toulouse (France) and as a Masters student at UI Urbana-Champaign, his training was primarily in ecology and evolutionary biology. During this time, he developed a keen interest in conservation biology that later led him to genetics. He completed his Ph.D. at North Carolina State University under the mentorship of Drs Eric Stone and Trudy Mackay. During his doctorate, he developed various approaches that centered on using a systems genetics approach to dissect the genetic basis of complex traits in Drosophila. He was then elected to the Harvard Society of Fellows as a Junior Fellow, where he studied the relationship between standing natural genetic variation and phenotypic variation, bridging theoretical and empirical approaches. His background in ecology and evolution grounds him as an organismal biologist, and it is in that context that he approaches the molecular and functional work in the lab.

Julie Peng
Research Specialist
Julie did her Bachelor in Medicine at Harbin Medical University, China and Ph.D. in Molecular and Cell Biology at SUNY-Downstate Medical Center under the supervision of Dr. Maureen McLeod. She studied signal transduction pathways regulating meiosis using fission yeast Schizosaccharomyces pombe as a model. Prior to joining the Ayroles lab, she worked as a research specialist in the Andolfatto lab at Princeton University. She applied various genomic technologies, especially Next Generation Sequencing (NGS) on understanding genome evolution and genetic mechanisms underlying adaptations in a variety of species. She is interested in developing novel genomic methods and high-throughput automation for large scale population genetic studies.

Scott Wolf
Graduate Student
Scott received his BS in mathematics along with minors in history and English from the University of Arkansas at Little Rock. As an undergraduate, he was involved extensively in software development in industry and academia. At Princeton, he is interested in how the foundations of mathematics, computer science, and statistics intersect with physiology, genomics, and neuroscience to give insight into complex biological systems.
Email
Benjamin Muhoya
EEB Graduate Student
Benjamin received his Bachelor’s in Medical Laboratory Science from the Technical University of Kenya and is now a PhD student in the Ecology and Evolutionary Department of Princeton University. He is interested in evolutionary medicine; and more specifically, he plans to focus on how evolutionary mismatch contributes to the development of cardiometabolic disease. Benjamin joined the Turkana Health and Genomics Project at the Mpala Research Center in Kenya in 2019. As part of the team, he focused on mapping out the prevalence of cardio-metabolic disorders among Turkana populations living in different locations and leading different lifestyles (i.e., subsistence lifestyle vs Urban). As a graduate student in the Ayroles Lab, Benjamin will continue working on cardiometabolic disorders, approaching this problem from an evolutionary perspective to determine the genetic and environmental factors that drive the variations in cardiometabolic among populations leading different lifestyles.

Charles Miano
Graduate Student
Charles has been a research scientist working with the Turkana Health and Genomics Project since 2021, in Kenya. He holds a Bachelor of Science in Biochemistry, with a major in medical research, and he is currently pursuing a Master of Science in Molecular Medicine from the Kenya Medical Research Institute, JKUAT School of Health Sciences, in Kenya. His research interests revolve around evolutionary medicine and genetics. Specifically, he is interested in exploring how environmental factors associated with lifestyle changes (e.g. rural-urban transition) interact with pathogen exposure or infections (e.g. soil-transmitted helminths) contribute to variation in immune function and dysregulation. To address this question, he combines in vivo and in-intro stimulation using field-collected PBMCs. He is joining the Ayroles lab at Princeton University as a visiting student research collaborator, where he will further pursue his interest.

Emmanuel D’Agostino
EEB Graduate Student
Emmanuel is an EEB graduate student studying speciation and adaptation in Drosophila using epistatic and polygenic frameworks. He is interested in understanding how the accumulation of genetic incompatibilities and changes in gene regulation within species drives speciation. Before coming to Princeton, he was a research specialist in Daniel Matute’s lab at the University of North Carolina-Chapel Hill, where he studied the role transposable elements play in speciation and the evolution of disease vectors. Emmanuel completed his AB at Harvard where he conducted undergraduate thesis work in Jonathan Losos’ lab on mating behavior in brown anoles, and worked as an undergraduate technician in Hopi Hoekstra’s lab. Outside of the lab, he enjoys running, hiking, reading, and rooting for sports teams from his hometown of Boston.
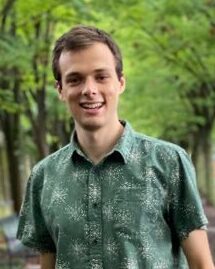
Diogo Melo
Postdoc
Diogo has an undergraduate degree in biology from the University of São Paulo, where he also obtained a master’s degree (2012) and a Ph.D. (2019) in genetics and evolutionary biology, working with Prof. Gabriel Marroig. Diogo’s work is centered on the evolution of genetic correlations, a topic he explores using several different approaches, including QTL mapping, experimental evolution, computer simulations, and comparative data. He joins the Ayroles lab as a Princeton Presidential Postdoctoral Fellow.

Kristina M. Garske
Postdoc
Kristina obtained her BS in biochemistry & cell biology and her MS in biology from the University of California, San Diego (UCSD), where she studied cardiovascular development in zebrafish with Prof. Deborah Yelon. She then moved to the University of California, Los Angeles (UCLA) to get her PhD in human genetics under the supervision of Prof. Päivi Pajukanta. She studied the genetics of cardiometabolic disorders and how context-dependent genomic regulatory mechanisms (gene-environment interactions) in adipose tissue cell types contribute to increased risk for obesity-related traits such as body mass index (BMI) and fat distribution, serum lipid levels, and inflammation. In the Ayroles Lab at Princeton, Kristina will continue to work in the gene-environment interaction space to understand how past exposure or adaptation to certain environments affects future responses to novel environments.

Cara Weisman
Postdoc
Cara was an undergraduate at Harvard, where she studied Molecular and Cellular Biology and Philosophy. She stayed at Harvard for her PhD in Biophysics, during which she studied where lineage-specific genes, which lack apparent homologs outside of a restricted group of species, come from and what they might do. She is now a Lewis-Sigler Scholar studying evolutionary novelty more broadly: what features of molecules and cells change during evolution, how fast does this happen, and what are the mechanisms underlying it?

Boniface Mukoma
Project Manager
Boni is the Project Manager. He is a self-disciplined, industrious, and resourceful scientist with eight years of experience in research. He leads our team through many challenges, which requires systematic implementation of project protocols as well as flexibility and creativity.

Francis Lotukoi
Logistics
Francis has been with the project from the very beginning and has been instrumental to getting this project off the ground. He is the lead driver for the project and so much more!
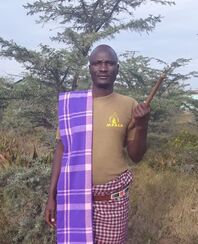
Echwa John
Research Assistant
Echwa is a Research Assistant who assists with community engagement, interviews, and data management. Echwa is from Rumuruti and speaks English, Turkana, Swahili, and Gikuyu. He is interested in how communities change through time and how this lifestyle change will affect future generations.
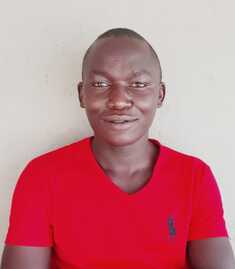
Anjelina Lopurudoi
Research Assistant
Anjelina is a Research Assistant who assists with translation and interviews as well as data management. Anjelina is from Juakali and speaks English, Turkana, Swahili, and Samburu.

Patriciah Waruguru
Research Scientist
Patriciah is a molecular biologist based at the Mpala research center in Kenya, serving as a Senior research specialist. working on the Turkana Health and Genomics Project. She is particularly interested in immunology and aims to understand how does an individual history of immune exposure influence pro-inflammatory response
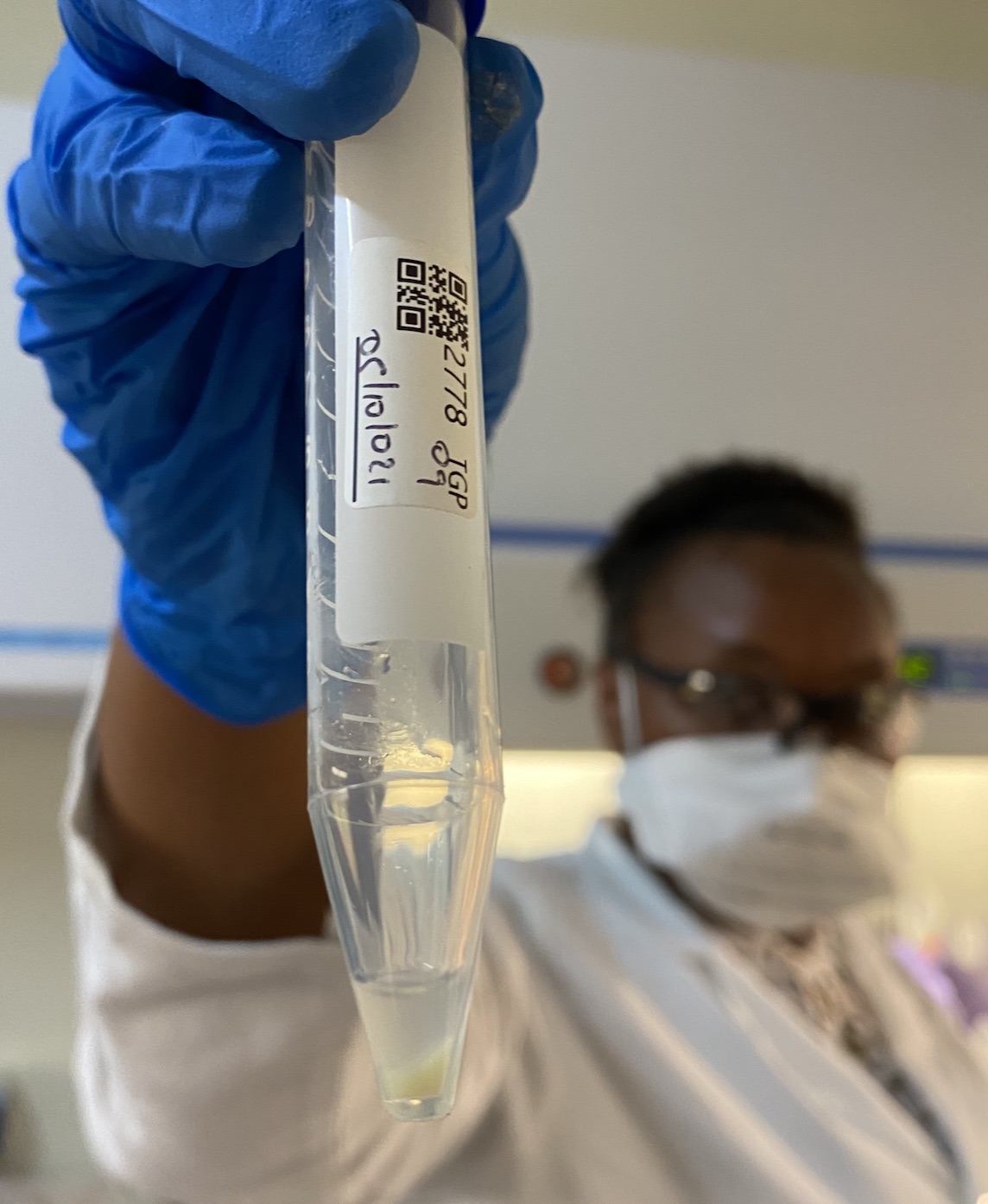
Peruse our publications
Published:
60- Pettie, KP, Mumbach, M, Lea, AJ, Ayroles, JF, Chang, HY, Kasowski, M, & Fraser, HB. (2022). Chromatin activity identifies differential gene regulation across human ancestries. Genome Biology, in press.
59- Pallares, L. F., Lea, A. J., Han, C., Filippova, E. V., Andolfatto, P., & Ayroles, J. F. (2023). Dietary stress remodels the genetic architecture of lifespan variation in outbred Drosophila. Nature genetics, 55(1), 123-129.
58- Melo, D., Pallares, L. F., & Ayroles, J. F. (2023). Reassessing the modularity of gene co-expression networks using the Stochastic Block Model. bioRxiv, 2023-05
57- Pallares, L. F., Melo, D., Wolf, S., Cofer, E. M., Abhyankar, V., Peng, J., & Ayroles, J. F. (2023). Saturating the eQTL map in Drosophila melanogaster: genome-wide patterns of cis and trans regulation of transcriptional variation in outbred populations. bioRxiv, 2023.05.20.541576.
56- Lea A, Clark A, Dahl A, Devinsky O, Garcia A, Golden C, Kamau J, Kraft T, Martins D, Mogoi D, Pajukanta P, Perry G,Pontzer H, Trumble B, Urlacher S, Venkataraman V, Wallace I, Gurven M, Lieberman D, Ayroles, JF. (2023). Applying an evolutionary mismatch framework to understand disease susceptibility. Plos Biology 21(9), e3002311.
55- Wolf, S*, Melo, D*, Garske, KM, Pallares, LF, & Ayroles, JF. (2022). Characterizing the landscape of gene expression variance in humans.PLoS genetics 19 (7), e1010833.
54- Lea, A. J., Caldas, I. V., Garske, K. M., Echwa, J., Gurven, M., Handley, C., … & Ayroles, J. F. (2023). Adaptations to water stress and pastoralism in the Turkana of northwest Kenya. bioRxiv
53- Lea, A. J., Garcia, A., Arevalo, J., Ayroles, J. F., Buetow, K., Cole, S. W., … & Gurven, M. D. (2023). Natural selection of immune and metabolic genes associated with health in two lowland Bolivian populations. Proceedings of the National Academy of Sciences, 120 (1) e2207544120
52- Lea, A. J., Peng, J., & Ayroles, J. F. (2022). Diverse environmental perturbations reveal the evolution and context-dependency of genetic effects on gene expression levels. Genome Research, 32(10), 1826-1839
50- Bruijning, M., Henry, L. P., Forsberg, S. K., Metcalf, C. J. E., & Ayroles, J. F. (2022). Natural selection for imprecise vertical transmission in host–microbiota systems. Nature ecology & evolution 6(1):77-87.
49- Henry, L. P., & Ayroles, J. F. (2022). Latitudinal cline does not predict variation in the microbiome of wild Drosophila melanogaster. Molecular Ecology 31(22), 5861-5871.
48- Henry, L. P., Fernandez, M., Wolf, S., & Ayroles, J. (2022). Wolbachia interacts with the microbiome to shape fitness-associated traits during seasonal adaptation in Drosophila melanogaster. bioRxiv.
47- de Bivort, B., Buchanan, S., Skutt-Kakaria, K., Gajda, E., Ayroles, J., O’Leary, C., … & Smith, M. A. Y. (2022). Precise quantification of behavioral individuality from 80 million decisions across 183,000 flies. Frontiers in Behavioral Neuroscience, 16.
46- Golden, C. D., Ayroles, J., Eurich, J. G., Gephart, J. A., Seto, K. L., Sharp, M. K., … & Timeon, E. (2022). Study Protocol: Interactive Dynamics of Coral Reef Fisheries and the Nutrition Transition in Kiribati. Frontiers in Public Health, 10:890381.
45- Bruijning, M., Ayroles, J. F., Henry, L. P., Koskella, B., Meyer, K. M., & Metcalf, C. J. E. (2022). Relative abundance data can misrepresent heritability of the microbiome. bioRxiv.
44- Werkhoven, Z., Bravin, A., Skutt-Kakaria, K., Reimers, P., Pallares, L.F., Ayroles, J. and de Bivort, B.L., 2021. The structure of behavioral variation within a genotype. Elife, 10, p.e64988.
43- Choi, J.Y., Dai, X., Alam, O., Peng, J.Z., Rughani, P., Hickey, S., Harrington, E., Juul, S., Ayroles, J.F., Purugganan, M.D. and Stacy, E.A., 2021. Ancestral polymorphisms shape the adaptive radiation of Metrosideros across the Hawaiian Islands. Proceedings of the National Academy of Sciences, 118(37).
42- Henry, L.P., Bruijning, M., Forsberg, S.K. and Ayroles, J.F., 2021. The microbiome extends host evolutionary potential. Nature Communications, 12(1):1-13.
41- Akhund-Zade, J., Lall, S., Gajda, E., Yoon, D., Ayroles, J.F. and de Bivort, B.L., 2021. Genetic basis of offspring number-body weight tradeoff in Drosophila melanogaster. G3 Genes| Genomes| Genetics.
40- Lea, A.J., Siford, R., Phung, T., Taravella, A., Lutukoi, F., Lowasa, S., Muhoya, B., Waigwa, C., Davidson, S., Handley, C. and Kamau, J., 2021, March. Uncovering the evolutionary history of the Turkana, a desert pastoralist group. American Journal or Physical Anthropology (174):60-60.
39- Lea, A.J., Waigwa, C., Muhoya, B., Lotukoi, F., Peng, J., Henry, L., Abhyankar, V., Kamau, J., Martins, D., Gurven, M. and Ayroles, J.F., 2021. Social gradients in health vary between rural and urban Turkana. Evolution, Medicine, and Public Health 9(1), 406–419.
38- Pallares, L.F., Lea, A.J., Han, C., Filippova, E.V., Andolfatto, P. and Ayroles, J.F., 2023. Diet unmasks genetic variants that regulate lifespan in outbred Drosophila. Nat Genet 55, 123–129.
37- Rau, C.D., Gonzales, N.M., Bloom, J.S., Park, D., Ayroles, J., Palmer, A.A., Lusis, A.J. and Zaitlen, N., 2020. Modeling epistasis in mice and yeast using the proportion of two or more distinct genetic backgrounds: Evidence for “polygenic epistasis. PLoS genetics, 16(10):e1009165.
36- Lea, A.J., Martins, D., Kamau, J., Gurven, M. and Ayroles, J.F., 2020. Urbanization and market integration have strong, nonlinear effects on cardiometabolic health in the Turkana. Science advances, 6(43):eabb1430.
35- Akhund-Zade, J., Yoon, D., Bangerter, A., Polizos, N., Campbell, M., Soloshenko, A., Zhang, T., Wice, E., Albright, A., Narayanan, A. and Schmidt, P., 2020. Wild flies hedge their thermal preference bets in response to seasonal fluctuations. Biorxiv (2020.09.16.300731).
34- Henry, L., Fernandez, M. and Ayroles, J.F., 2020. GxGxE interactions and adaptive potential of the microbiome in Drosophila melanogaster. bioRxiv (2020.09.21.306779).
33- Henry, L.P. and Ayroles, J.F., 2021. Meta-analysis suggests the microbiome responds to Evolve and Resequence experiments in Drosophila melanogaster. BMC microbiology, 21(1):1-14.
32- Bruijning, M., Metcalf, C.J.E., Jongejans, E. and Ayroles, J.F., 2020. The evolution of variance control. Trends in ecology & evolution, 35(1):22-33.
31- Pallares, L.F., Picard, S. and Ayroles, J.F., 2020. TM3’seq: a tagmentation-mediated 3’sequencing approach for improving scalability of RNAseq experiments. G3: Genes, Genomes, Genetics, 10(1):143-150.
30- A J Lea, M Subramaniam, A Ko, T Lehtimäki, E Raitoharju, MikaKähönen, I Seppälä, N Mononen, O Raitakari, M Ala-Korpela, P Pajukanta, N Zaitlen, Ayroles JF. (2019). Genetic and environmental perturbations lead to regulatory decoherence. eLife 2019;8:e40538
29- S Musharoff, DS Park, A Dahl, JM Galanter, X Liu, S Huntsman, C Eng, Burchard EG, Ayroles JF *, Zaitlen N* (2018) Existence and implications of population variance structure. bioRxiv, 439661 (*equal contribution)
28- Schrider DR, Ayroles JF, Matute DR, AD Kern AD. (2018). Supervised machine learning reveals introgressed loci in the genomes of Drosophila simulans and D. sechellia. PLoS genetics 14 (4), e1007341.
27- Dumitrascu B, Darnell G, Ayroles JF, Engelhardt BE. (2018). Statistical tests for detecting variance effects in quantitative trait studies. Bioinformatics 1, 11.
24 – Zwarts L, Broeck LV, Cappuyns E, Ayroles JF, Magwire MM, Vulsteke V, Clements J, Mackay TF, Callaerts P. (2015) The genetic basis of natural variation in mushroom body size in Drosophila melanogaster. Nature communications.11:6.
23 – Ayroles JF, Buchanan SM, O’Leary C, Skutt-Kakaria K, Grenier JK, Clark AG, Hartl DL, de Bivort BL. (2015). Behavioral idiosyncrasy reveals genetic control of phenotypic variability. Proceedings of the National Academy of Sciences 112(21):6706-11.
21 – Matute DR*, Ayroles JF*. (2014) Hybridization occurs between Drosophila simulans and D. sechellia in the Seychelles archipelago. Journal of evolutionary biology. 27(6):1057-68.
20- Corbett-Detig RB, Zhou J, Clark AG, Hartl DL, Ayroles JF . (2013). Genetic Incompatibilities Within Species are Widespread. Nature, 504, 135–137.
19- Huang W, Richards S, Carbone MA, Zhu D, Anholt RRH, Ayroles JF, et al. (2012) Epistasis Dominates The Genetic Architecture Of Drosophila Quantitative Traits. PNAS, 109:15553-15559.
18- Massouras A, Waszak SM, Albarca M, Hens K, Holcombe K, Ayroles JF, Dermitzakis ET, Eric A Stone EA, Jensen J D, Mackay T.F.C, Deplancke B. (2012) Genomic Variation And Its Impact On Gene Expression In Drosophila Melanogaster. Plos Genetics. 8 (11): e1003055.
17- Mackay TFC*, Richards S*, Barbadilla A *, Stone EA*, Ayroles JF*, Zhu D, Sònia Casillas. et. al. (2012) The Drosophila Genetics Reference Panel: A Community Resource for Analysis of Population Genomics and Quantitative Traits. Nature, 482(7384):173-8. Faculty of 1000, Biology
16 – Ober U, Ayroles JF, Stone EA, Richards S, Zhu D,Gibbs RA, Stricker C, Gianola D, Schlather M, Mackay TFC, Simianer H. (2011) Using Whole Genome Sequence Data to Predict Quantitative Trait Phenotypes in Drosophila melanogaster. PLoS Genetics, 8(5): e1002685. Faculty of 1000, Biology
15 – Rowe K, Singhal S, MacManes M, Ayroles JF, Morelli TL, Rubidge E, Bi K, Moritz C (2012). Museum Genomics: Low Cost And High Accuracy Genetic Data From Historical Specimens. Molecular Ecology Ressources, 11(6): 1082–1092.
14 – Ayroles JF, Laflamme B, Wolfner MA, Mackay TFC. (2011) Sifting Through The Data: Identifying Top Candidates For Novelseminal Protein Genes From Drosophila Whole Genome Expression Data. Genetics Research, 93(6): 387-395.
13 – Jumbo-Lucioni P*, Ayroles JF*, Chambers MM, Jordan KW, Leips J, Mackay TF, De Luca M. (2010) Systems Genetics Analysis Of Body Weight And Energy Metabolism Traits In Drosophila Melanogaster. BMC Genomics, 11(11): 297. (* Contributed equally)
12 – Edwards, A, Ayroles JF, Stone EA, Mackay TFC. (2009) A Transcriptional Network Associated With Natural Variation In Drosophila Aggressive Behavior. Genome Biology, 10(7): R76.
11 – Mackay TFC, Stone EA, Ayroles JF. (2009) Quantitative Genetics: Prospects And Challenges. Nature Review Genetics, 10(8): 565-577.
10 – Morozova TV*, Ayroles JF*, Jordan KW, Duncan LH, Carbone MA, Lyman RF, Stone EA, Govindaraju DR, Ellison RC, Mackay TF, Anholt RR. (2009) Alcohol Sensitivity In Drosophila: Translational Potential Of Systems Genetics. Genetics, 183(2): 733-745 (* Contributed equally)
9 – Harbison ST, Carbone MA, Ayroles JF, Stone EA, Lyman RF, Mackay TFC (2009) Co-Regulated Transcriptional Networks Contribute to Natural Genetic Variation in Drosophila Sleep. Nature Genetics, 41(3): 371-375.
8 – Ayroles JF, Carbone MA, Stone EA, Jordan KW, Lyman RF, Magwire MM, Rollman SM, Duncan LH, Lawrence F, Anholt RH, Mackay TFC. (2009) Systems genetics of complex traits in Drosophila melanogaster. Nature Genetics, 41(3): 299-307. Faculty of 1000, Biology
7 – Kocher SD, Ayroles JF, Stone EA, Grozinger CM. (2009) Genomics Of Pheromone Response: Cooperation And Conflict In Honey Bees. Plos ONE, 5(2): e9116.
6 – Stone EA, Ayroles JF. (2009) Modulated Modularity Clustering As An Exploratory Tool For Functional Genomic Inference. PLoS Genetics, 5(5): e1000479.
5 – Ayroles JF, Hughes KA, Reedy MM, Rodriguez-Zas SL, Drnevich JM, Rowe KC, Cáceres CE, Paige KN. (2009) Genome-Wide Assessment Of Inbreeding Depression In Drosophila Melanogaster. Conservation Biology, 23(4): 920-930.
4 – Carbone MA, Ayroles JF, Yamamoto A, Morozova TV, West SA, Magwire MM, Mackay TF, Anholt RR. (2009) Overexpression Of Myocilin In The Drosophila Eye Activates The Unfolded Protein Response: Implications For Glaucoma. PLoS ONE, 4(1): e4216.
3 – Ayroles JF, Gibson G. (2006) Analysis Of Variance Of Microarray Data. Methods Enzymol, 411: -33.
2 – Hughes KA, Ayroles JF, Reedy MM, Drnevich JM, Rowe KC, Ruedi EA, Cáceres CE, Paige KN. (2006) Segregating Variation In The Transcriptome: Cis Regulation And Additivity Of Effects. Genetics 173(3): 1347-1355.
1 – Dejean A, Solano PJ, Ayroles JF, Corbara B, Orivel J. (2005) Insect Behaviour: Arboreal Ants Build Traps to Capture Prey. Nature, (434):973.
Book Chapter:
1- Metcalf CJE*, Ayroles JF*. (2019). Chapter: “Why does intra-genotypic variance persist?” In book titled “Unsolved Problems in Ecology’. Princeton University Press. (*equal contribution)